Killers, cardinals, quarantines, jellybeans and vaccines
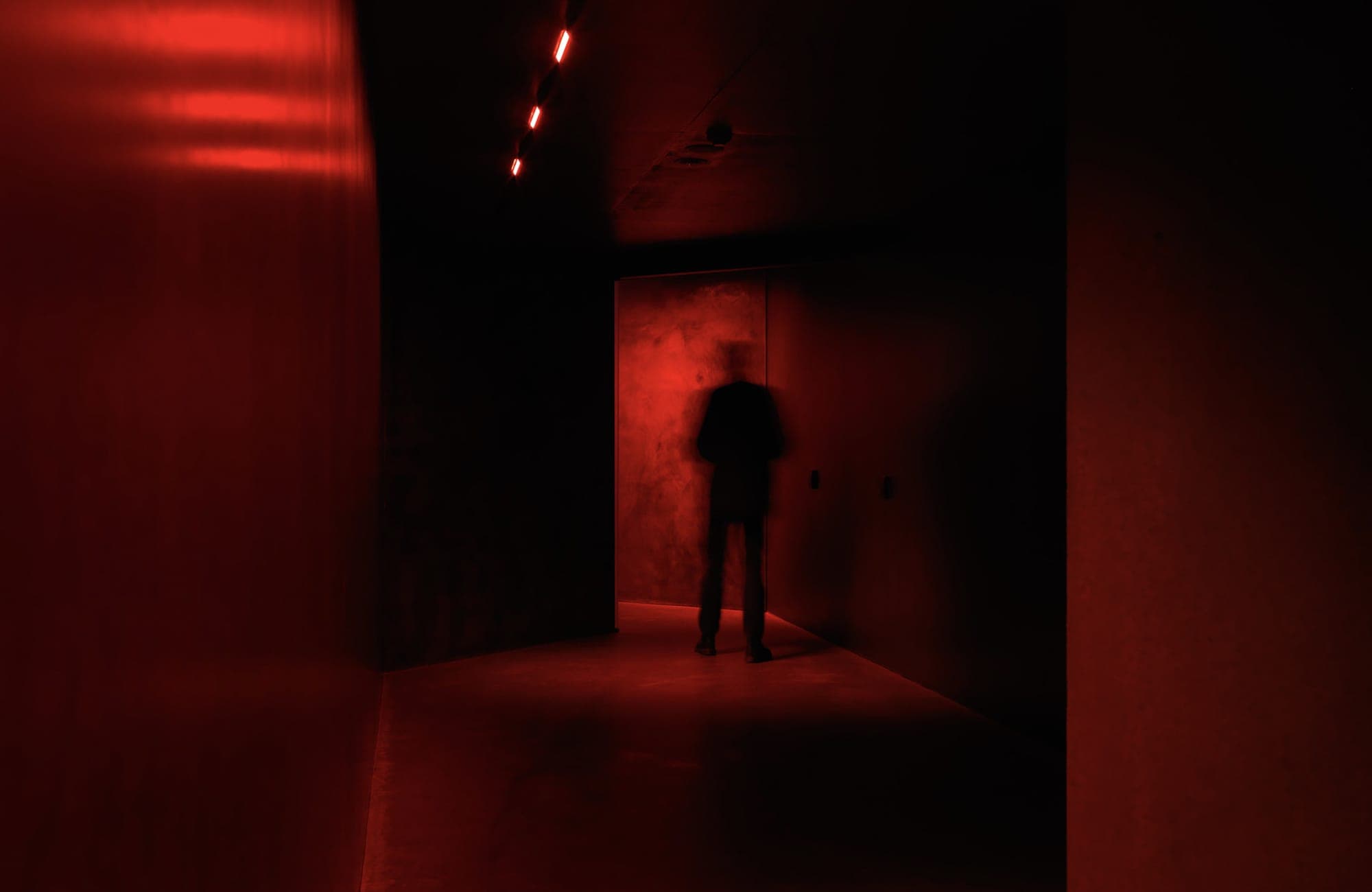
Monday 6–Thursday 9 April 2020
My mates take quarantine very seriously. That might be because they have a background in gambling, so they understand that unlikely events can have serious consequences. Or it might be because even miscreant gamblers rally round the flag in times of trouble (when the going gets tough, the tough stay put). Or it might be because, at various times, the police, the State Emergency Service, and the fire brigade have checked up on them (one young lady sequestered at Mona regretted that body contact isn’t permitted when a particularly considerable constable established her whereabouts).
In many locales, the checking up is done technologically. I thought the authorities would ask to befriend you on your phone (find-a-friend), so they’d know if you were roaming. But mostly, they send text messages that demand a locating response. That could be a little problematic. A small percentage of COVID-19 sufferers die suddenly, so the phrasing would have to be carefully considered. ‘We’d like to know where you are, and if you are alive’. Or, ‘If deceased, please disregard this message.’
But, eventually, quarantine finishes. For the quarantinas and quarantinos, nothing much changes. They move out of purgatory, into limbo. Limbo is not so bad. My daily (government-approved) walks are a highlight. There’s a new passing technique—if you have the road to the left you must swerve slightly onto it—and everybody compensates for the impoliteness of evasive manoeuvres with verbal greetings. My wife thinks these breathy salutations are a potential source of infection, but she is unable to maintain her silence when passing Africans from fear that her COVID terseness will be interpreted as racism.
And this is the new normal—it already seems normal. Sunday expects to greet her classmates online, Kirsha expects to cook, we expect to see a ‘carnival of animals’ on the Mona lawn, and I expect to be dismayed by the John Hopkins coronavirus dashboard (which is dismaying despite improvement at a rate I did not foresee). I also expect to spend my mornings blogging about things I barely understand.
That last will have to change. This is going to be my last blog for a while. Most locales are showing improvement; social distancing seems to be reducing R to less than one; Australians, at least, seem to be dutifully following directions; and I don’t have much more to say.
There will be more blogs from me. Unexpected events are inevitable when exponentials are in play, because outcomes are extremely sensitive to initial conditions. China is relaxing social restrictions. That’s an interesting natural experiment that I’m sure other polities will observe carefully. Can we re-engage without blowing exponential disease bubbles? Anyway, here’s the real reason I’m curtailing my blogging. My wife’s description of her life:
Play with kid.
Cook.
Clean.
Watch husband write blog.
Repeat.
I need to alleviate her groundhog days, while we await the edge of tomorrow.
But I’ve not finished this blog yet. In fact, I’ve barely begun.
Since I’m going away, I want to give you access to my decision-making toolbox (don’t apply anything you might learn here to betting—I need your money).
When is a decision a bad decision?
It’s not a bad decision when it contradicts the one you would have made. You’re as likely to be wrong as anybody else. You think you are a better than average driver. But almost everybody thinks they are a better than average driver. If you are a professor, you think that you are a better than average professor. But almost all professors think they are better than average professors.
You think you remember things well. You don’t. You think you remember important events more accurately than unimportant events. You don’t. A modest test: think about what you were doing when 9/11 occurred. Then check the time, and check with the people you think you were with.
Because memory is flawed, and a consensus is more likely to be accurate than you are, seek consensus opinion (under symmetric circumstances—everybody gets asymmetries wrong in the same way, and I’ll get to that). Don’t seek consensus from likeminded people. They’ll just confirm your opinion. Boards shouldn’t comprise the most talented group of people, they should be the most diverse group of people. Heterosexual couples face novel situations with more optionality than two random individuals would. A corollary is that men and women aren’t the same. They are different by design.
It’s not a bad decision just because outcomes show that there was a more productive pathway. The quality of a decision can only be assessed with information that was available at the time the decision was made (however, it’s a bad decision to not seek to maximise information—forget Malcolm Gladwell’s bullshit Blink). I’m a successful gambler. However, becoming a gambler was a bad decision, because the chance of me being successful (rich, attentive audience, Mona-induced status) was very small. The chance of me becoming addicted and destroying my life was much larger. Although I’m successful, it was a very bad decision to become a gambler. Most of the input cohort of potential gamblers didn’t turn out like me, and before events ran their course, there was no way to discriminate between me and them. Judging my decision to be a gambler to be a good decision requires data that was unavailable at the time the decision was made. If you still think I must be skilled, given how well stuff turned out for me, ask yourself this: what set of skills qualified Donald Trump to be the author of the fate of the United States?
It’s not a bad decision when it fails to conform to your ideology. That means that the rules can’t be framed in such a way as to drive a particular ideology. You don’t like that your rules don’t rule the world. Neither did Hitler. A world conforming to your ideals is not a utopia. Not even for you. The world will not conform to your standards even if it tries to. And it’s a really bad idea to garner all your information and opinions from those that agree with you. If you’re a lefty, find some anti-immigrant mates, and watch Fox News. And if you lean right, hang around with barrackers for abortion, and read The Guardian.
Here’s a little test (called the Wason Selection Task):
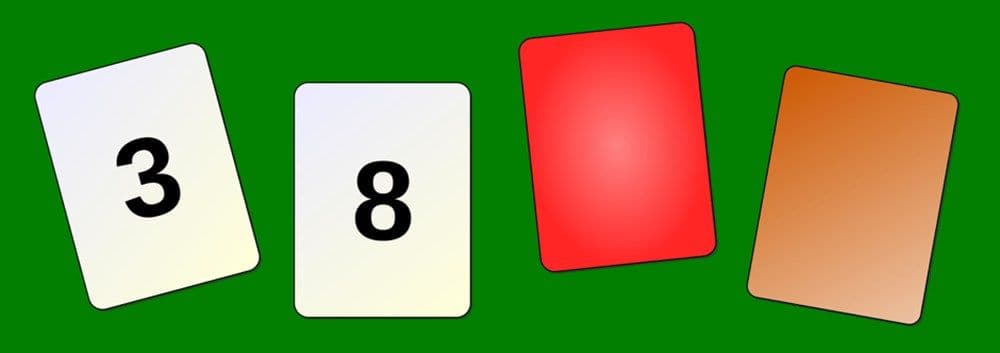
Each card has a number on one side, and a patch of colour on the other. What card or cards must be turned over to test the idea that if a card shows an even number on one face, then its opposite face is red?
Did you turn over the 8 card, and the brown card? Turning over the 3 card doesn’t test anything, because the statement didn’t assert anything about the colour of odd cards. And turning over the red card doesn’t help, either, because although you need to know if even is red, you don’t need to know if red is even.
Did you seek to falsify the hypothesis, or confirm it? Confirming data doesn’t prove an idea is true. But contradictory data will show it’s false. So try to understand why those who disagree with you, disagree with you. Don’t seek data that conforms with your beliefs. And don’t interpret data in such a way that it confirms your beliefs. Also, don’t ignore data that contradicts your beliefs (those are examples of confirmation bias—your tendency to favour information that strengthens your beliefs).
It’s not a bad decision because it’s made by someone you tend to disagree with. In fact, someone you tend to disagree with is more useful to you than someone you agree with, as long as you are actively seeking consensus. Because, most likely, they will interpret data differently than you do.
Without evidence, don’t align yourself with the opinion that your foes are likely to disagree with. I see lefties thrashing about trying to find reasons to castigate Morrison concerning his handling of COVID-19. It’s the cruise ships, or what he’ll do when it’s all over, or the safety net isn’t wide enough (or too wide). When criticising decisions made by natural enemies, there is a strong tendency to evaluate those decisions with data compiled after the decision was made.
It’s a bad decision if it expands (or ignores) tail risk. This is a big one. The most consequential strategic mistakes fall through the chasms that tail risk opens. But it needs some explanation.
Firstly, if you are trying to win at the races, do you try to pick winners? If you think the answer is yes, tail risk will kill you. Consider the roll of a single fair die. There is a one in six chance that it comes up on a particular number—say, four. If you place a bet, you are purchasing an amount in the event that a four comes up. This game is a good game, and each time a four comes up you are paid ten dollars. A four comes up one time in six, and you therefore receive ten dollars one time in six. During a representative sequence of six bets (or, over a long period of time, when the law of large numbers determines that the ratio of rolled fours to total number of rolls gets closer and closer to one in six), you will have five losses and one win. If you are betting one dollar per roll, you will lose five dollars and have one win that returns ten dollars. Thus, having bet six dollars for a five-dollar profit, a margin of 5/6 or 0.833—an 83.3 per cent profit. Each roll, on average, is a purchase of a chance of four coming up, and costs one dollar with sell price $1.83. It’s a business transaction. Except that most games that offer a bet on dice rolling, and they are more convoluted bets than my example, don’t return a $1.83. They return $0.98 or so. If you got the average every time, nobody would buy. Nobody would pay one dollar to acquire $0.98. But the games are sufficiently complicated that cognitive biases can set in. In the short term, a gamble seems unpredictable, because the gambler doesn’t see it as a purchase of the average return (the expectation). They see it in terms of an individual outcome. And that, as I said, allows (or forces) them to exhibit their biases. Having an advantage (an edge) over a sequence of events is called a statistical arbitrage. This is because a simultaneous buy/sell with an advantage is called an arbitrage. A business transaction with the sell price already set is exactly the same thing, in my opinion. And a statistical arbitrage, a sequence of bets with an edge, is only different because it is an average.
Now, a horse race is a die roll, with unequal sides. In a given race, one horse might have one chance in ten of winning, so the payout needs to be more than $10 for it to be an advantage. If it’s paying $11, it’s a 10 per cent advantage. Now here’s another horse in the same race. This one is one chance in two of winning, so it’s five times more likely to win then the other horse. But it’s paying $1.80, so the return for $1 is $0.90. Just as in the example before, it’s a business transaction, and each time you engage you are spending $1 to acquire $0.90. The horse with five times better chance of winning is a bad bet, the long shot is a good bet.
It’s often possible to evaluate horserace odds so that the odds you bet with are better than those of the public, or the bookmaker. Evaluating probabilities in the real world is often not like that. So let me use the stock market as an example. You might well suspect that the market is going to go up nine times out of ten, so if you bet the market is going to go up you win, right? Not necessarily. The one time in ten it goes down, it might go down more than enough to make you lose more than all the times it went up. It’s similar to the horse race, but without the odds being quantified.
Now consider a lottery. They are bad bets, only about one half of the money wagered is returned to the punters (expectation 50%, ostensibly a bad business decision). There might just be one chance in five million that you win a million dollars, but a million dollars might be worth more than five million times a dollar to you. The risk is worth taking for you because you have an asymmetry in your exposure to risk. That’s a positive asymmetry. Now consider a negative asymmetry. If you are rational and it can kill you, you don’t take risks. If I offer you a game of Russian roulette, five blanks, one deadly bullet, and each blank pays you a million dollars, you won’t play that game. Even though you have five chances to get rich for one chance to get dead, you won’t play that game. The negative asymmetry is untenable.
And from society’s point of view negative asymmetries are even more undesirable. Just one thousand-to-one shot can fuck us all up. If we keep taking on negative asymmetric risk, we are doomed. But we, as individuals and as societies, tend to behave as if unlikely things don’t happen.
To reiterate: if, for example, the COVID-19 deniers are likely to be correct (‘it’s just like the flu’), say ninety-five per cent likely, then should we do nothing? Of course not: this is one outcome in a sequence of potential disasters. We only need take fourteen gambles of this type before our chance of suffering a disaster is greater than fifty per cent. After taking fifty-nine such risks, the chance of one potentially inopportune occurrence is greater than ninety-five per cent.
Now let’s apply the principle that emerges from this (it’s called the Precautionary Principle) to global warming. Firstly, there is debate about the reality of global warning, but a consensus has emerged that suggests that only the scale is unknown (you might think it’s crap, but in any case, remember that opinions you don’t agree with are more valuable to you). If global warming is real, the downside, the asymmetry, is untenable. It doesn’t matter if it’s likely. Even if it’s very unlikely, but possible, it’s a bad bet, and we have to intervene. While scientists and politicians argue whether it’s real, the intervention, which must happen, becomes more costly. We have to bet bigger for the same return.
Should we let COVID-19 cavort through the community? No. It might be flu-level harmless (it probably isn’t). But without intervention, it initially expands exponentially. Its effects, if there are effects, compound quickly. So should we throw the economy under a bus to suppress COVID-19? Abso-bloody-lutely. It must be suppressed, so until we know the minimum cost to suppress it (other countries are helping, with a range of natural experiments), we have to do whatever it takes. The asymmetry demands it.
And we would be throwing the economy under a bus, anyway. Those who assert that the price paid by the economy is too big are comparing the shit we are in with how it was when everything was hunky dory. But without government intervention everything still would have changed. If there had been no intervention for COVID-19, restaurants and bars wouldn’t be full. As fatalities increased people would intervene to protect themselves, and they’d choose to stay at home. And they certainly wouldn’t be flying, or taking cruises (having learnt a lesson, they may never take cruises again, so I do hope that governments don’t bail out cruise companies). In other words, economies would still be collapsing, but there would be lots more dead people, and services would be massively overloaded.
Up until now, all of the asymmetries we’ve discussed have been forecastable (or, at least, within the bounds of reason). But most things that generate massive change aren’t forecastable. While we wait for flying cars (an easy extrapolation of existing technologies), we get the internet, and Facebook. COVID-19 isn’t an unforecastable risk (in fact it was well known that some type of pandemic was inevitable, eventually). As discussed before, the risks that can’t be forecast are called black swans (https://www.dymocks.com.au/book/the-black-swan-by-nassim-nicholas-taleb-9780141034591). Black swans are one of the reasons that we expect less unlikely events than we actually get (fat tails). But, again, COVID-19 wasn’t a black swan. Black swans, by definition, can’t be forecast, so we need a range of different strategies to deal with them. Mostly, we should run with positive asymmetries, and limit our losses with negative asymmetries. That works for all asymmetries, including black swans. For negative black swans in particular, we should preserve resources at a societal level, but more importantly, we should organise societies locally, and then with naturally emerging groupings. Federalism is occasionally required, but only when it emerges from the sum of increasingly overarching local scales. The best social organisation preserves a property called scale invariance. That’s hard to explain, except mathematically, but consider a coastline. If you look up how long a coastline is, you’ll get an answer. I just did for the coastline of Australia, and Google told me it’s 25,760 kilometres. But that’s bullshit. If the yardstick is 1000 km (yes, I see the irony), then the coastline is about 15000 km. If the yardstick is a millimetre, and every rock on the foreshore is counted, then it’s millions of kilometres. And if the yardstick is the size of an atom, it’s vastly bigger again. But, without the yardstick, zooming in or out, the coastline looks the same. That’s the property of scale invariance, and that’s a property we should be preserving for our social and political organisation. If we want goods made well, we need local scale artisanship. If we want resources that exist in only a few domains (lithium, for example, to power my iPad that’s powering my rant), we need a larger scale, but it should look the same if we zoom out. Efficiency suffers with scale invariance, monocultures are replaced (in part) with permacultures, and large-scale mass production is replaced with scaled industry. Resources and products become more expensive, debt is scaled across polities, protectionism is enabled through tort law. But robustness and flexibility are embedded in the system. It won’t fail, globally.
Ok, I went a bit far with that for a first lesson. Take this home. If you want a system to be robust, don’t give it points of failure. Scale it so that at each scale there are other, perhaps less efficient, pathways to fulfilling the same need. If Australia survives this calamity, or another that we can’t forecast, and Europe and America doesn’t, we haven’t really survived at all. That’s a diagnostic that shows that the system is failing. If we want our societies to survive over scale-invariant time, then they need to be robust against all scaled impactors, across all domains.
Elon Musk wants a sustainable colony on Mars. That makes sense, the Earth is a single point of failure, and there are some impactors (giant meteorites, runaway global warming), that can wipe us out. If he’s right, though, then responding at all scales is also right. And Musk is no oracle. On 7 March he tweeted, ‘The coronavirus panic is dumb.’ But not panicking, when faced with an existential threat, however unlikely to rescale—that’s dumb. This was poor judgement, with information at the time it was made (and long before).
I’ll try one more tack before I move on. If you ask people to guess how many jellybeans there are in a jar, the more people you ask, the better the estimate. Statistical methods are useful for providing insights like how many people will provide what sort of accuracy.
Take those same people and ask them to trade jellybean futures. The price won’t be bound to supply and demand, and the errors won’t simply come from inaccurate enumeration of supply and demand. They’ll be gluts, and booms, and busts, and hoarding and runs in the market. This is because there is feedback in the system. Opinions affect price points because they constrain demand or corral supply, but those opinions also affect others’ opinions, and those opinions also affect prices.
When trading jellybean futures, statistics don’t work. Things will happen that have never happened before. The variance may be infinite, and forecasting fraught. But there will still be a few that get rich, and they’ll get rich together. They’ll think they are very clever, and maybe join forces to form a club promoting the free market, and jellybean future trading.
So what’s the feedback that makes a system difficult to forecast? It’s a magnification of an input, like a recording feeding into a microphone. COVID-19 populations would be hard enough to forecast anyway, since exponential effects produce a vast array of outcomes from tiny differences in initial conditions, but with attempts to dampen down the system (stay home, keep your distance, don’t breathe) the system becomes unstable. And the scale of the dampeners varies according to their success. Today (9 April) The Australian quotes a bunch of business leaders who are advocating for a swift reopening of the economy. That might generate another exponential bubble, or it might not (and you know by now that we should behave as if it will).
A characteristic of unforecastable systems is fat tails. Unlikely events happen much more often than the normal distribution would indicate that they should (so that if you plot a bar graph of the frequency of events, you get more than expected outcomes at either end—fat tails).
If you attempt to forecast extreme events, fat tails will always bite you on the bum. Recently Parisian authorities have been planning measures to respond to the Seine flooding, after two big floods in three years. They are planning for the highest levels so far encountered (in 1920). They’ll get a bigger flood, eventually. And Australia just had ‘unprecedented’ bushfires. They’ll have them again. And this pandemic, we’ll have one that’s more ‘pan’. And some bad thing that we have no insight into (a black swan) will come along that’s worse (and some other thing that’s better), than anything that’s happened so far.
If you try to control jellybean prices by increasing demand (eating them), or by increasing supply (buying a packet wherever it is that you can’t get toilet rolls), the price will behave in an unstable way. There’s a lesson there for OPEC, and for closed economies, and for open economies. You won’t get what you want, ‘and if you try sometimes, you just might find’, you don’t get what you need, either.
It’s a bad decision if it ignores sensitivity testing. Right now, a bunch of models are being applied to forecast outcomes for COVID-19. They can be useful, because they might detect phenomena that otherwise aren’t obvious. But, because of their sensitivity to initial conditions and systemic assumptions, they don’t forecast anything. If Australia had kept its coronavirus-positive exponential growth rate, it would have detected 45,000 positive people by now. There are about 6,000. Very slight changes in parameters that produce dramatic outcomes (and those parameters are mostly below the resolution of the models), produce vastly different forecasts. So should Australia have planned for 6,000, or 45,000? Neither. It should have planned for a population-wide infection, the very unlikely worst case (and, for the most part, it did). This is chess against a formidable opponent. Don’t plan for a mistake, plan for the best move that opponent can make. But at least, when you do your modeling, put in a whole bunch of values across a range of assumptions, so that you know whether your model forecasts the same thing every time. If it does, your model might be useful. But mostly, it won’t.
As I was thinking about this the Australian Government published a description of the models they had employed (prepared by the Doherty Institute)1. They made significant assumptions.
Transmission parameters were based on information synthesis from multiple sources, with an assumed R0 of 2.53, and a doubling time of 6.4 days... Potential for pre-symptomatic transmission was assumed within 48 hours prior to symptom onset.
Those assumptions are not tested with sensitivity analysis. Since these factors are not known with certainty, the model should be run over a range of values for each of them (some sampling was conducted on the severity of illness). Only if the forecast is the same in all these test cases, can policy be based on forecasting. Because this was not done, policy should be based on the worst-case scenario (and that, creditably, seems to be how the Government formulated its policy).
It’s a bad decision if it commits to an invariant path. There’s an article in The Age today (8 April), arguing that Australia should decide what its long-term strategy for COVID-19 is.2 That’s extremely dangerous. Firstly, whether we choose to reduce our social isolation isn’t a decision that needs to be made, as yet. In all situations we should preserve our optionality as long as possible. In this situation the path is the same, for a while, whatever the most appropriate option, so why choose now? Secondly, the article specifies mutually exclusive options as if they are mutually exclusive, and as if it’s impossible that other options will arise. We have made extreme social changes that are apparently working. Let’s stay the course to see if the apparent improvements are real. And let’s observe all the polities that are implementing other strategies. Those natural experiments can teach us a great deal. They might show us a way that The Age columnist didn’t foresee. It’s at least possible.
We are waiting for a miracle vaccine, and discussing timelines shorter than the development of any vaccine so far. But it’s not hopeless. The disease might evolve to be less lethal, or a treatment might be found, or a black swan might appear. What black swan? I don’t know. That’s the point. If we keep our options open, we recruit the unpredictable to our corner.
It’s a bad decision if it increases degrees of freedom without compensatory systemic functionality. If you are doing something okay, perhaps modelling horseracing in New Zealand and winning, or building houses out of bricks, then you don’t make changes to improve the system, unless the improvements are simple, incremental, and substantial. Break losing systems by all means (only slightly), but preserve things that are going right. A revolution replaces an apparently dysfunctional system—but with what? A new system, that’s had no sensitivity testing, and didn’t derive from incremental understanding. It’ll be worse. There are more configurations of a system that break it than make it better. To make a change, find an improvement that’s worthy, and stress test the system under the new regime. Programmers (at least good ones) know that it’s risky to fix a bug that doesn’t matter. Fixing bugs often creates new bugs. But even that is better than starting from scratch.
I wrote down a bunch of other rules. But if I keep writing I’ll disincentivise you from reading anything I write that you have to pay for. More importantly, I’ll incentivise my wife to find another life. If you want to know more, you shouldn’t be reading a blog, anyway. You should be reading Nassim Taleb.
While I’m pontificating on important stuff I know nothing about let me pontificate on the pontiff’s prefect, Cardinal Pell. Lowlife, perhaps. Elitist, indulgent, self-righteous prig. Obdurate enabler. Probable pedophile. But guilty based on the testimony of one decent man, who is recounting a memory of his boyhood? Because he was a decent man? Pell was not guilty beyond a reasonable doubt. And the system didn’t fail the jurors, nor did it fail justice. It error-checked it, and thus enhanced the merit that the whole process possesses. I enjoyed seeing Pell squirm. But should the impartiality of jurisprudence be abandoned for every naughty boy, or girl? For me? Or you?
I had a moment with the law. A strange moment, when I thought the law and I were on divergent paths. I was fifteen—particular, superior, insular, alone. A young lady with whom I hardly interacted tried to remedy the latter two categories. Despite my urgent need, I had no idea what she was offering, that her offering was herself. I rejected her, without knowing. And then, on 4 September 1977, an old lady was murdered.
My suitor, my potential paramour, was interviewed by the police. She, rejected and apparently dejected, described the culprit—me. The police tore my life apart. For a couple of months I attended grade eleven under suspicion of murder. I don’t think I was particularly fazed. I assumed justice would prevail.
It did. Rodney Wayne Williams was convicted of the murder of Dorice Rodman and, eventually, served eighteen years. He was released in 1995.
Eight days after my daughter Sunday was born in 2015, sixteen-year-old, pregnant Tiffany Taylor was murdered in southern Queensland. Neither she, nor her unborn child, were availed the opportunity to grow up and ponder the injustice of their circumstances. And on the day that a fleet of military vehicles conveyed COVID-19 victims from Bergamo to crematoria outside of the district of Lombardy, Rodney Wayne Williams was convicted of his second murder. Whether through evil, or disability, or alteration through incarceration, Mr Williams visited on Dorice and Tiffany a thing, and that same thing was impartially delivered by evolutionary concoction to those casualties in Lombardy, and many others being flung about our fragile bubble.
Somehow, being returned to the crimes of Rodney Williams reignited my compassion for those who have perished without the impartial application of justice in this calamity. Dorice and Tiffany, those names I know; their lives and the lives of the anonymous others are composed of the moments preceding; and their deaths, the moments curtailed. Much damage is done by the adventitious marauding of statistical process. Mr Williams is both much more, and much less, than a virus.
1 https://www.doherty.edu.au/uploads/content_doc/McVernon_Modelling_COVID-19_07Apr1_with_appendix.pdf
2 https://www.theage.com.au/national/now-the-lucky-country-must-decide-what-is-our-least-worst-option-on-covid-19-20200403-p54gq8.html